Generative AI vs Machine Learning: The Differences Explained
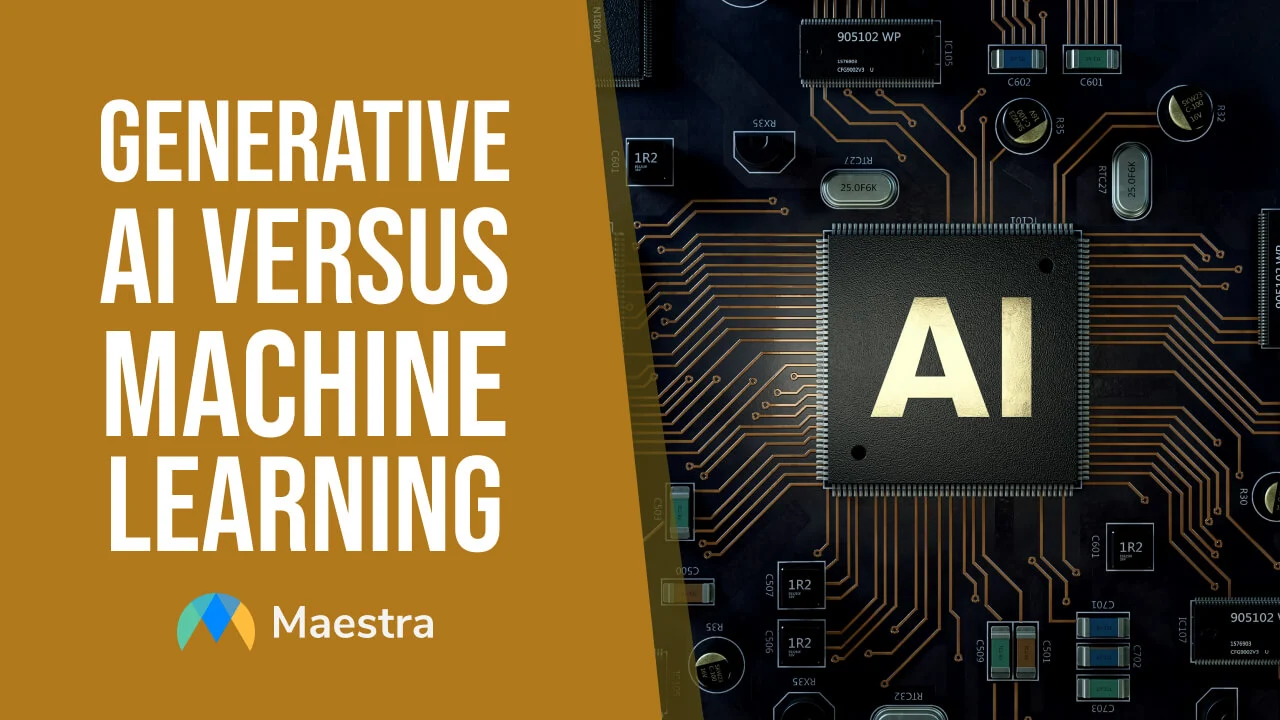
AI has been reshaping every aspect of our lives, and we come across new terms each passing day. Generative AI also known as GenAI and machine learning (ML) are the two most popular of those terms. Their popularity makes generative AI vs machine learning a subject of discussion.
In this blog post, we will explain generative AI and machine learning and compare the two AI models in terms of their use cases, core processes, and limitations.
Generative AI vs Machine Learning: A Table of Comparison
Aspect | 🔮 Generative AI | 🤖 Machine Learning |
---|---|---|
🌠 Primary Goal | Generating new content | Pattern recognition, classification, and decision-making |
🛠️ Output | Creative | Predictive |
⚙️ Core Processes | Data collection and training
Learning patterns Content creation Fine-tuning and feedback |
Data collection and preprocessing
Feature selection Model training Prediction Optimization |
➕ Pros | Creative and innovative outputs
Adaptable to various industries |
Accurate predictions and classifications
Wide range of applications |
➖ Cons | Resource-intensive
Risk of creating unethical content (e.g., deepfakes) Possible lack of contextual understanding Hallucinations |
Requires large amounts of labeled data
Possible performance issues with unseen data Computationally intensive Ethical concerns in biased data predictions |
🧰 Applications | Content creation (blogs, videos, etc.)
Art and design (DALL·E, etc.) Voice synthesis (Maestra AI, etc.) Game development (creating characters, etc.) Drug discovery simulations |
Fraud detection
Forecasting (weather, sales, etc.) Recommendation engines Healthcare diagnostics Autonomous vehicles |
What is Generative AI?
Generative AI is an artificial intelligence model that is able to produce creative outputs. It creates new content based on the training data.
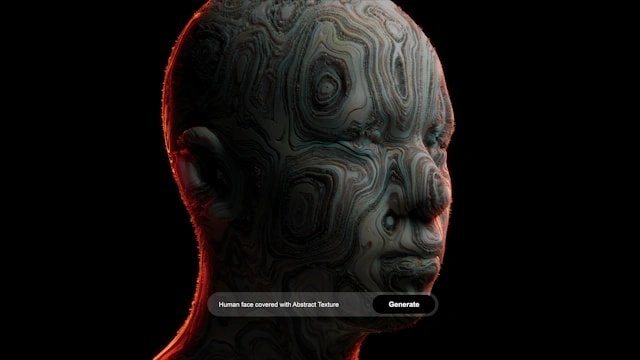
This model is frequently used for text generation, creating visuals, voice synthesis, and game development. The most common examples are ChatGPT for text generation, DALL·E for AI art, and Maestra AI for voiceovers.
Technologies:
What is Machine Learning?
Machine learning is a predictive AI model. With ML, machines can analyze data and make predictions and decisions based on them, even if they weren’t primarily programmed to make that decision.
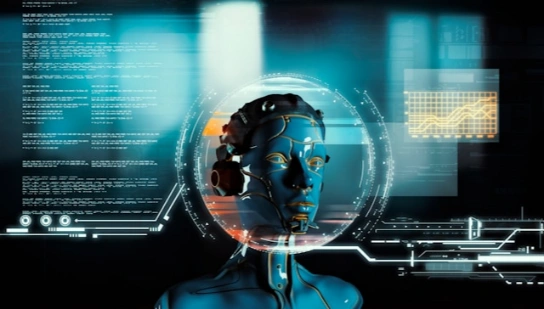
This model is frequently used in recommendation engines, banking, healthcare, e-commerce, autonomous vehicles, and natural language processing. The most common examples are the recommendation systems of Netflix and Spotify, fraud detection in banking, and disease diagnosis from medical scans.
Technologies:
Generative AI vs Machine Learning: Differences Explained
Since they are two different models, they have different purposes, processes, applications, outputs, and challenges.
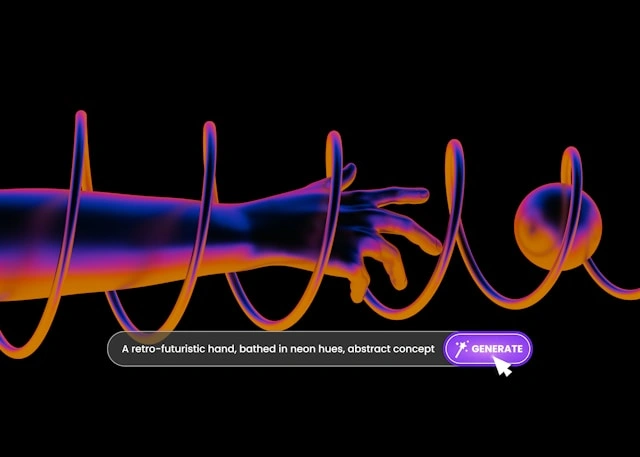
Core Processes
AI systems follow certain steps to perform tasks. These steps change depending on the type of the task or AI model.
Generative AI
Machine Learning
Challenges and Limitations
Another important point of comparison about generative AI vs machine learning is their disadvantages. These two models use different technologies and function using different processes, so there are different challenges that comes with each AI model.
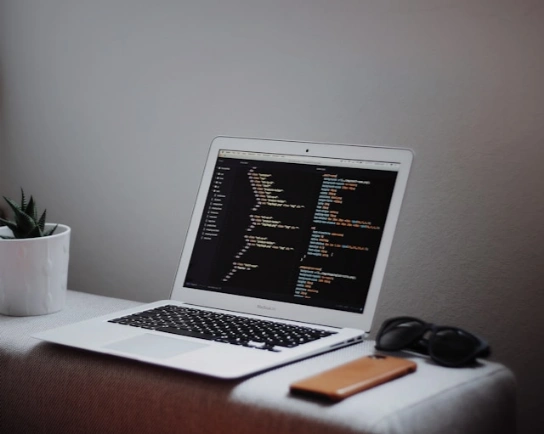
Generative AI
Machine Learning
Applications
Another difference between generative AI and machine learning is their applications. Generative AI can be used to create new content, edit existing content, and create animations. Machine learning, on the other hand, can be used for predictive analytics and automation. Let's take a closer look at how we use GenAI and ML in our daily lives:
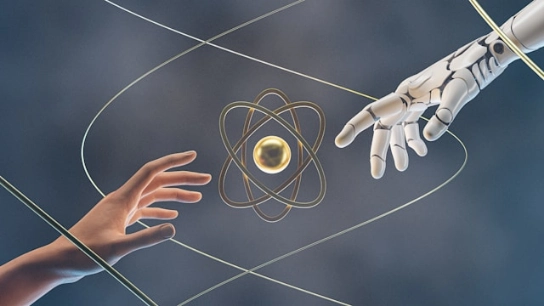
Generative AI
Machine Learning
Combining GenAI and Machine Learning
Even though generative AI and machine learning are different AI models, it is not possible to say that they are completely unrelated.
Generative AI models are trained using machine learning too, and there are many applications using both models like Maestra.
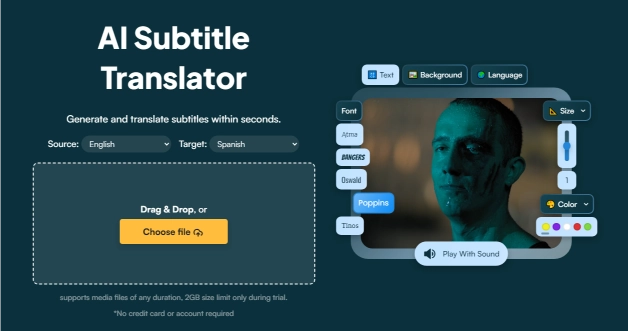
Maestra AI offers a suite of tools enabling you to generate transcripts, subtitles, and voiceovers in real-time or on demand.
See machine learning and generative AI at work.
Create subtitles and generate voiceovers in 125+ languages using Maestra.
Frequently Asked Questions
Is machine learning the same as generative AI?
No. machine learning and generative AI are different AI models. While machine learning focuses on recognizing patterns among the given dataset and making predictions, generative AI creates new content using machine learning.
Is ChatGPT AI or machine learning?
Both. ChatGPT is an AI chatbot powered by machine learning technology that creates text outputs. It mimics human-like interactions, and since it generates text, it is a generative AI model.
Will generative AI replace machine learning?
While it seems like generative AI is getting more and more popular each day, we cannot say that it will replace machine learning because generative AI itself uses machine learning. Even though generative AI works differently, it needs machine learning techniques to function.
Will generative AI replace developers?
Generative AI can help developers automate tasks, write documentation, debug, and come up with solutions, so developers can benefit from generative AI to enhance their productivity. But, skills like critical thinking, designing system architectures, and understanding the specifications of certain tasks are unique to people. That’s why human oversight is essential for ensuring accuracy, quality, and security while coding.